As AI evolves, effective collaboration of the entire project life cycle is still a difficult task for the AI team.
In fact, 20%of the AI leaders are not satisfied with the need for collaboration, emphasizing that cohesive AI teams are as essential to build the AI itself.
As AI initiatives increase complexity and size, organizations that promote strong and cross -functional partnerships have an important advantage in competition for innovation.
This fast guide strengthens the collaboration between teams with AI leaders and has a practical strategy to ensure a softer workflow, faster progress and more successful AI results.
Teamwork obstacles AI leader is facing
AI collaboration is a team silver, changing the working environment, and increasing the wrong sorted goals and business demands.
For the AI team, these tasks appear in four major areas.
- split: Due to separate tools, workflows and processes, the team is difficult to operate with a cohesive device.
- Coordination complexity: Depending on the size of the project, adjusting the cross -function team for handoff priorities, timelines and dependencies becomes exponential.
- Unconscious communication: The gap between communication is to miss the opportunity and cause confusion about redundancy, re -work and project status and responsibility.
- Model integrity: To ensure model accuracy, fairness and security, smooth handoffs and continuous supervision are required, but teams that have been disconnected have a lack of observation tools for shared liability or maintenance.
Solving these obstacles is important for AI leaders who want to simplify their operations, minimize risks and pursue meaningful results faster.
Sculpture workflow, tools and languages
The AI project usually passes five teams, seven tools and 12 programming languages before reaching a business user. This is just the beginning.
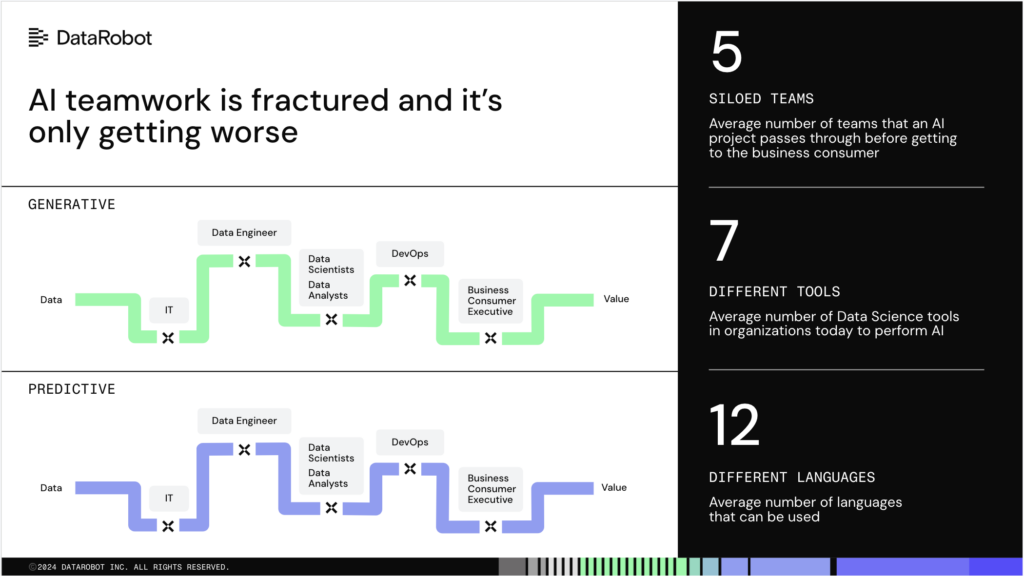
Here’s how fragmentation interferes with collaboration and what you can do to solve the AI leader.
- Separated project: Silo between teams creates Oh Jung -ryul. Design a clear workflow and sharing goal at the planning stage.
- Cloned effort: Duplicate work slows progress and creates waste. To avoid overlapping, use shared documents and centralized project tools.
- Completion delay: Bad hand off creates bottlenecks. Implementing a structured handoff process and adjusting the timeline to keep moving the project.
- Tools and coding language compatibility: Incompatible tools interfere with interoperability. Standardize as much as possible to improve compatibility and simplify collaboration.
If the process and team are fragmented, it is more difficult to maintain a unification vision for the project. Over time, these Oh Jung -ryul can erupt the business impact of the final AI output and the participation of user.
Hidden handoff cost
Each stage of the AI project presents a new handoff, which has a new risk for progress and performance. The situation is often wrong.
- Data gap from research to development: Incomplete or inconvenient data transfer and data replication are slowly developed and re -work increases.
- Missed expectationThe unclear test criteria lead to defects and delays during development vs. test handoffs.
- Integration problemThe difference in the technology environment can cause failure if the model moves from the test to the production.
- Weak monitoringA problem that is not detected due to limited directors after distribution can harm model performance and endanger business operations.
To alleviate these risks, AI leaders must synchronize the cross -function team at each development stage to preserve the amount of project exercise and provide a solution that guarantees a more predictable and controlled batch path.
Strategic solution
Team communication breaks down the barrier
AI leaders are increasingly disabled in simplifying workflows to increase efficiency while combining code priority and low code teams. This disconnection is important, citing cooperation between teams when 13%of AI leaders develop AI use cases through various lifetime periods.
To solve these tasks, AI leaders can focus on two key strategies.
1. Provides context for adjusting the team
The AI leader plays an important role in making the team understand the entire project context, including the youth case, business relevance, intended results and organizational policies.
Integration of these insights into approval workflow and automated guard rails maintains clarity of roles and responsibilities, protects sensitive data such as personal identification information (PII), and ensures policy compliance.
By prioritizing transparent communication and including a context in the workflow, the leader creates an environment where the team can confidently innovate without risk of sensitive information or operational integrity.
2. Use the centralized platform for collaboration
The AI team requires a centralized communication platform to collaborate in the model development, testing and distribution stage.
Integrated AI Suite can simplify the workflow by allowing the team to tag assets, add opinions, and share resources through the central registry and youth case hub.
Major functions, such as automated versions and comprehensive documents, provide clear historical records, simplify handoffs, and track the project and ensure work integrity.
The AI leader combines clear context settings with centralized tools to relieve team communication gaps, eliminate redundancy, and maintain efficiency in the entire AI life cycle.
Model integrity protects from development to distribution
For many organizations, models are used 7 months or more It reaches production regardless of AI maturity. This long timeline introduces more opportunities for errors, inconsistencies and wrongly sorted goals.
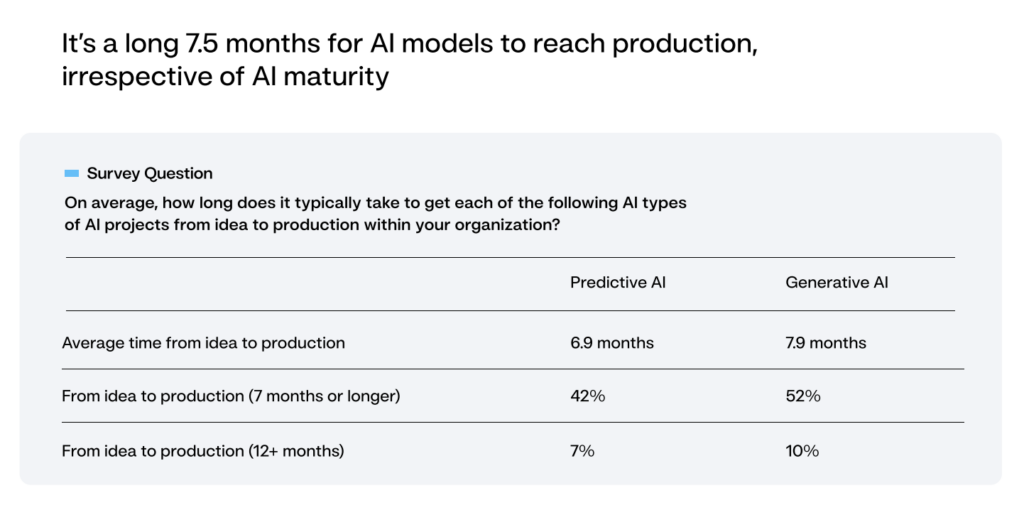
AI leaders are as follows to protect model integrity.
- Automate documents, versions and histories.
- In all stages, invest in technologies with custom security guards and deep observation.
- It gives the AI team to make the model easy and consistently test, verify and compare.
- Provide collaboration work space and centralized hub for smooth communication and handoffs.
- Set well monitored data pipelines to prevent drifts and to maintain data quality and consistency.
- Emphasize the importance of model documents and perform regular audits to meet the standards of compliance.
- Set a clear criterion for the timing of the model update or maintenance and develop a rollback strategy that can return to the previous version if necessary.
By adopting these practices, AI leaders can guarantee, reduce risks, and provide influential results.
It is leading the way of AI collaboration and innovation
As an AI leader, you have the power to create an environment in which collaboration and innovation thrive.
By promoting sharing knowledge, clear communication, and solving group problems, the team can motivate and focus on high influential results.
For deeper insights and executable guidelines, we reveal how to explore our unbearable AI request reports and strengthen AI strategies and team performance.
About the author
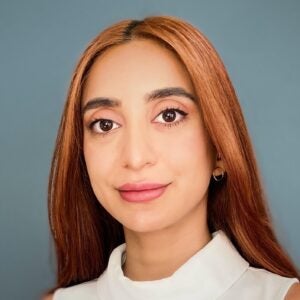
May masoud is a data scientist, AI advocate and thinking leader. In Datarobot, she designs a market strategy of Datarobot AI Governance to help global organizations derive measurable profits for AI investment while maintaining corporate governance and ethics.
MAY developed the Technology Foundation through statistics and economic degrees and developed a master’s degree in business analysis of the Schulich School of Business. Cocktails of this technology and business knowledge have formed May as an AI practitioner and thinking leader. You can democratize the AI keynote and workshops for ethical AI and business and academic community.
May meet in May