Generative AI grabs the headlines, but the reality is that most AI use cases today that create measurable business value are predictive.
We recently released 22 new features that help extend our predictive AI solutions and ensure model integrity and performance from build to deployment.
Today we’re going to look at some new improvements in DataRobot that will help you quickly prepare data for modeling and evaluate model performance when building predictive AI models.
💡Pro Tip: Use DataRobot to build custom projects that leverage the combined power of predictive AI and generative AI to achieve new levels of innovation and impact.
Enhanced AI data preparation for model accuracy and performance
There are few steps more tedious than transforming and preparing your data for modeling. At DataRobot, we’ve always made it easy to get your dirty data ready for AI, and we make it easy for you. With DataRobot, you don’t have to drag and drop to prepare your data before modeling, just point DataRobot to a file or table, and the platform handles the rest. Now, we’ve added all the great features you know and love about AI data preparation in the Classic UX, to the new NextGen interface.
Secure Data Connection: Easily find, share, and leverage your data with enhanced navigation and preview capabilities, and profile details in NextGen’s cloud data warehouse, cloud storage, and AI catalog.
Wrangle, Join and Aggregate: Improve your data workflows by seamlessly combining, aggregating, and transforming data directly from your supported cloud data warehouse or from your data stored in DataRobot AI Catalog and Blob storage. Point DataRobot to a table (or tables), quickly identify signals in your data, and easily materialize that data into your data warehouse for reuse in NextGen.
Feature discovery: DataRobot has always been unique in the way it does feature engineering and feature discovery. Now, you can access all these rich features and build recipes for specific use cases to create new datasets with features derived from NexGen.
💡Pro tip: If you’re using the SaaS version of DataRobot, you already have access to these new features in the latest version of DataRobot. If your organization uses an on-premise solution, you’ll need to manually update DataRobot to see the latest and greatest improvements.
AI-driven insights and explainability at your fingertips
Explainability is essential to building trust in a model. Whether you’re providing AI-based recommendations or advocating for productionization of a model, being able to interpret how the model works and makes decisions is a critical capability.
Explainability is not only essential for driving model adoption from business stakeholders, but also for understanding key drivers of outcomes and gaining deep AI-driven insights. how and why When your model works, you can create more powerful changes within your organization. We’ve expanded and added these insights to NextGen UX.
Explaining Predictions with SHAP Insights: Quickly understand your predictions with improved SHAP explainability support for all model types and a new individual PE feature that computes SHAP values for each row.
Slice Insight: View and compare insights based on segments of your project data to gain a better understanding of how your models perform across different subsets. Slice your data by date/time, numeric, categorical, and Boolean data types.
Easily compare and optimize models
The latest capabilities in Workbench make it easier than ever to train and compare a variety of predictive models in DataRobot. You can quickly select experiments and evaluate key performance indicators, and integrate new insights into the NextGen UI to quickly understand model effectiveness and improve performance. We’ve also started the process of migrating all of the multi-model capabilities available in Classic UX to NextGen, starting with Time Series.
Enhanced confusion matrix: Train a classifier on a dataset with unlimited classes in Workbench, then quickly understand the effectiveness of the classifier with an enhanced confusion matrix.
Side-by-side modeling insights: Easily evaluate model performance across experiments using different data sets and modeling parameters, and quickly improve model performance by comparing models across multiple experiments.
Time series experience: Build powerful, detailed time series forecasts easily in the new NextGen UX and explore the new features added.
Unified view of notebook and non-notebook files
For code-first users, we’ve invested significant resources into delivering a best-in-class experience. In this release, we’ve improved Codespaces to allow you to open, view, and edit multiple notebook and non-notebook files simultaneously, so you can focus on building models rather than infrastructure. New improvements make it easier to edit and run files, and develop new workflows.
Codespaces and Codespace Scheduling: Build reusable, automated workflows with new Codespace features. Open, view, edit, and run multiple notebook and non-notebook files in the same container session. Easily set up automated jobs at any desired interval. Monitor scheduled notebook jobs and track execution history. Develop automated, reusable workflows that can be easily executed by configuring scheduled notebooks.
Nearly infinite scale in modeling and inference time
As data continues to explode, the amount of data your team works with every day is growing exponentially. This new release gives you the ability to work with larger datasets for builds and inferences, and does so in a very efficient way.
The ever-increasing cloud costs are starting to become a challenge for AI teams that need to balance effective training with budget constraints. Since our founding in 2012, DataRobot has focused on helping data science teams maximize their investments. In this case, we do this by not charging based on consumption, unlike most AI and data platforms that have an incentive to increase cloud costs. Our latest release further increases the value of your efforts by giving your teams the freedom to work with big data without worrying about costs.
Scale improvement: Seamlessly handle large datasets throughout the ML lifecycle with incremental learning and improved NVIDIA GPU compatibility. Our incremental learning is designed to get you the best model without spending time processing all your data. It also alerts you when more data is being used and returns are diminishing, so you don’t waste time modeling.
💡Pro Tip: Easily move your projects and datasets to the latest DataRobot environment with extended project migration. To get the most out of new features, visual elements, and collaboration capabilities, use the following:
Features designed to convey influence
While GenAI gets a lot of attention, we know many of you are having significant success with predictive AI. Our latest release shows that DataRobot continues to invest in predictive AI, while many other AI vendors are chasing the hype cycle and leaving predictive AI products aside. We know that true impact requires a combination of predictive and generative, and DataRobot is where AI teams go to deliver real results for their businesses.
Our customer community continues to discover new use cases and mature existing AI initiatives with incredible momentum. The average number of projects per customer has increased 12% year-over-year, and forecasts have increased 11% per customer.
The latest DataRobot enhancements give you more control over the critical early development phase. But the innovation doesn’t stop there. Stay tuned for a deep dive into Summer Launch ‘24, where we’ll look at recently introduced features that simplify how you deploy, observe, and manage your predictive models.
About the author
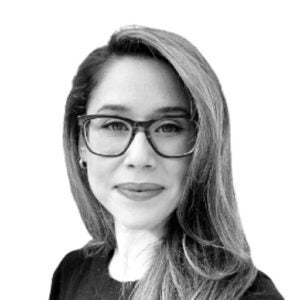
Lisa Aguilar is DataRobot’s VP of Product Marketing and Field CTO, responsible for building and executing the go-to-market strategy for the company’s AI-driven predictive product line. She works closely with the product management and development teams to identify key solutions that address the needs of retailers, manufacturers, and financial services providers using AI. Prior to joining DataRobot, Lisa worked at ThoughtSpot, a leader in search and AI-driven analytics.
Meet Lisa Aguilar